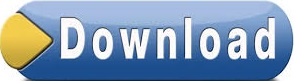

There have been several recent attempts to produce automatic and objective gates. For these reasons manual gating has become a major limiting aspect of flow cytometry, and there is a widely recognized need for more advanced analysis techniques. It is subjective as it is based on the user's interpretation and experience, it is error-prone, difficult to reproduce, time consuming, and does not scale to a high-throughput setting. There are several obvious problems with this kind of analysis. The cells falling in this gate are extracted and the process is repeated for different 2D projections of the gated cells, thus resulting in a sequence of two-dimensional gates that describe subpopulations of the multivariate flow cytometry data. The current standard technique for this type of analysis is to draw 2D gates manually with a mouse on a computer screen, based on the user's interpretation of density contour lines that are provided by software tools such as FlowJo ( ) or BioConductor.

One important part of the analysis of flow cytometry data is gating, that is, the identification of homogeneous subpopulations of cells. A major road block to tapping the full potential of this technology is the lack of data analysis methodology and software that allows for an automated and objective analysis of the data generated by this high-throughput instrument. This ability has made flow cytometry a prevalent instrument in both the research and clinical settings. Introductionįlow cytometry allows to measure simultaneously multiple characteristics of thousands of cells. We illustrate the methodology with a sample of mouse spleen and peritoneal cavity cells.

Our approach is nonparametric and can reproduce nonconvex subpopulations that are known to occur in flow cytometry samples, but which cannot be produced with current parametric model-based approaches. Our approach advances the paradigm of manually gating sequential two-dimensional projections of the data to a procedure that automatically produces gates based on statistical theory. We describe methodology and software to automatically identify cell populations in flow cytometry data. A current limit to the potential of this technology is the lack of automated tools for analyzing the resulting data. Made this technology ubiquitous and indispensable in the clinical and laboratory setting. The ability of flow cytometry to allow fast single cell interrogation of a large number of cells has
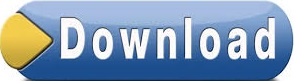